Predicting Groundwater Contamination from 1,2,3-TCP in California
Groundwater is a critical water source for many areas of California, especially in dry years when it contributes up to 60% of statewide water supplies [1]. Communities across the state rely on groundwater for drinking and essential household needs, with an estimated 83% of Californians using groundwater for at least a portion of supplies [2]. Groundwater is often a clean and reliable water source, but when groundwater is polluted, it can pose health risks, especially for small communities that rely on private groundwater wells.
While many groundwater pollutants in California are extensively monitored, for some pollutants, the extent of contamination and possible treatment solutions are not well understood [3]. One such contaminant is 1,2,3-trichloropropane (TCP), a legacy pollutant that was used in agricultural soil fumigants. Groundwater contamination from TCP is especially challenging in areas such as the Central Valley and Central Coast of California, where communities located close to agricultural fields rely on groundwater. Additionally, testing for TCP in water is costly, and many wells remain untested. However, recent research at UC Merced, published in the Journal of Groundwater for Sustainable Development [4], shows how machine learning can improve our understanding of where TCP may have accumulated. Below, we examine the historical roots of TCP contamination and explore how this new research may help communities address this persistent challenge.
Industrial Chemicals and Farming
After World War II, technological advancements led to widespread use of industrial chemicals, including pesticides with chlorine and fluorine. These chemicals were highly effective at controlling pests, but some are very persistent, meaning they remain in the environment for a long time without breaking down and pose health risks. TCP entered the environment as an impurity in soil fumigant pesticides that were designed to protect a plant’s root system from nematodes. Chemical companies were aware of the presence of TCP in the fumigants, but farmers were not. Although TCP was removed from pesticide formulations in the 1980s, the chemical lasts multiple decades before breaking down, so its legacy continues to impact California's groundwater.
In 2017, the California State Water Resources Control Board adopted a water quality standard for TCP in drinking water with a Maximum Contaminant Level (MCL) of 5 parts per trillion (ppt) [5, 6]. In areas where TCP levels exceed this amount, communities face health risks. Studies link TCP to cancer in animals, and it is believed to pose similar risks to humans [7]. Many private domestic wells have not been tested, leaving the full extent of TCP contamination unclear and some residents unaware of potential exposure.
Forests and Trees
How extensive is TCP contamination in California? In the absence of extensive monitoring data, our research used machine learning models to predict the accumulation of TCP in the Central Valley Aquifer System—a key groundwater source for California and a region deeply affected by TCP contamination due to its agricultural activity. Among the machine learning models tested, a method called Random Forest [8] proved to be the most accurate at predicting TCP levels in the Central Valley. Think of a Random Forest as a group of trees that each grew a unique way - much like a collection of flowcharts each with many paths. Each tree (flowchart) independently makes a prediction based on a different subset of data. Results of the individual trees are averaged together and, by combining these individual predictions, the model (forest) arrives at a more accurate conclusion than any single tree could.
We mapped our model’s final predictions across the Central Valley to highlight potential contamination hotspots (see figure below). The model predicts that higher concentrations of TCP are more frequent in the San Joaquin Valley and the Tulare Basins compared to the more northerly Sacramento Basin. Based on modeling, TCP contamination disproportionately affects regions with a greater number of disadvantaged communities. These impacts emphasize the need for low-cost treatment solutions.
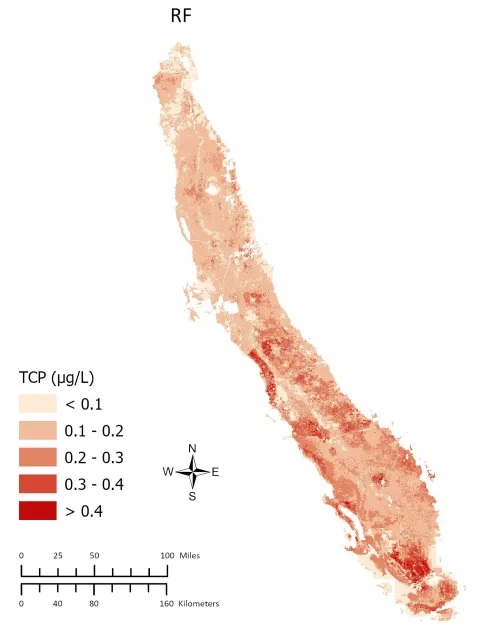
Modeling predicted groundwater 1,2,3-trichloropropane (TCP) concentrations using Random Forest.
In addition to mapping hotspots, we identified key factors that influence contamination. These include irrigation and precipitation patterns, nitrate levels and redox state. Redox state refers to the balance between oxygen-rich (oxidizing) and oxygen-poor (reducing) conditions in groundwater, which can affect how chemicals break down or persist over time. The results suggest that contamination risk is highest in years with more irrigation and less rainfall during times after TCP usage. These conditions increase the risks that water carrying undiluted TCP infiltrates deeper into the ground. In addition, groundwater with elevated nitrate levels, often found in agricultural areas, tends to also have higher TCP concentrations, as both contaminants leach into the groundwater together. Finally, since TCP degrades more slowly in oxygen-rich environments, groundwater with higher levels of dissolved oxygen tends to carry higher levels of TCP compared to groundwater with less oxygen. These findings increase our knowledge of contamination factors and suggest opportunities for applying the model in regions with limited or no TCP monitoring.
From Models to Action
While machine learning is a valuable tool to predict where TCP might be found, it is just one piece of the puzzle. Our study shows the need for equitable remediation efforts, since disadvantaged communities are at greatest risk of impacts from contamination in California. Currently, affected areas rely on costly solutions like installing and maintaining large granulated activated carbon (GAC) filtration systems [9] or blending contaminated water with cleaner sources to meet regulatory standards. However, these solutions can strain smaller water systems and leave domestic well owners shouldering high testing and treatment costs.
California has ambitious goals to ensure the human right to safe and affordable drinking water for all [10]. Small communities and properties reliant on private groundwater wells need additional information and resources to secure safe drinking water. We need to develop both stronger outreach and education programs to help well-owners access existing water testing resources, as well as more affordable small-scale testing and treatment technologies to meet their needs. State and regional agencies also have an important role in supporting research and funding programs for groundwater remediation efforts in the most affected areas, including offering technical assistance for infrastructure upgrades. Understanding how TCP contamination risk varies through modeling could help pinpoint priority areas for education and outreach. These insights can transform data into targeted guidance for California water managers, but modeling alone isn't enough—on-the-ground knowledge and testing remain essential.
Hope Hauptman is an Assistant Project Scientist with the California Institute for Water Resources. She is a former member of the FEW-US Lab at UC Merced, where she conducted research on TCP and groundwater contamination.
Acknowledgments: Thank you to Drs. Erik Porse and Colleen Naughton for their input to this article.
-----------------------------------------------------------------------------------------------------
Research that this article is based on: LINK
Hauptman, B. H., Naughton, C. C., & Harmon, T. C. (2023). Using machine learning to predict 1,2,3-trichloropropane contamination from legacy non-point source pollution of groundwater in California's Central Valley. Groundwater for Sustainable Development, 22, 100955. https://doi.org/10.1016/j.gsd.2023.100955
References
[1] Ortiz-Partida, J. P., Weintraub, C., Fernandez-Bou, A. S., & Maskey, M. L. (2020). Climate change in the San Joaquin Valley: A household and community guide to taking action. Union of Concerned Scientists. https://doi.org/10.13140/RG.2.2.36113.86882
[2] California Department of Water Resources. (n.d.). Groundwater. https://water.ca.gov/water-basics/groundwater/
[3] Tomich, T. P., Brodt, S. B., Dahlgren, R. A., & Scow, K. M. (Eds.). (2016). The California nitrogen assessment: Challenges and solutions for people, agriculture, and the environment. University of California Press. ISBN: 978-0520287129
[4] Hauptman, B. H., Naughton, C. C., & Harmon, T. C. (2023). Using machine learning to predict 1,2,3-trichloropropane contamination from legacy non-point source pollution of groundwater in California's Central Valley. Groundwater for Sustainable Development, 22, 100955. https://doi.org/10.1016/j.gsd.2023.100955
[5] U.S. Environmental Protection Agency. (2017a). Technical fact sheet – 1,2,3-Trichloropropane (TCP). https://www.epa.gov/fedfac/technical-fact-sheet-123-trichloropropane-tcp
[6] California State Water Resources Control Board. (2017). Groundwater fact sheet: 1,2,3-Trichloropropane (TCP). https://www.waterboards.ca.gov/gama/docs/coc_tcp123.pdf
[7] Tardiff, R. G., & Carson, M. L. (2010). Derivation of a reference dose and drinking water equivalent level for 1,2,3-trichloropropane. Food and Chemical Toxicology, 48(6), 1488–1510. https://doi.org/10.1016/j.fct.2010.03.016
[8] Breiman, L. (2001). Random forests. Machine Learning, 45, 5–32. https://doi.org/10.1023/A:1010933404324
[9] Hauptman, B. H., & Naughton, C. C. (2021). Legacy 1,2,3-trichloropropane contamination: A systematic review of treatments. Journal of Water, Sanitation and Hygiene for Development, 11(4), 515–534. https://doi.org/10.2166/washdev.2021.006
[10] California Legislature. (2012). Assembly Bill 685: Human right to water. https://leginfo.legislature.ca.gov
-----------------------------------------------------------------------------------------------------
Header image credit: "Pesticide application for chemical control of nematodes in a sunflower planted field" by Zeynel Cebeci, retrieved from: https://commons.wikimedia.org/wiki/File:Pesticides_application_03.jpg#Licensing, licensed under [Creative Commons License].